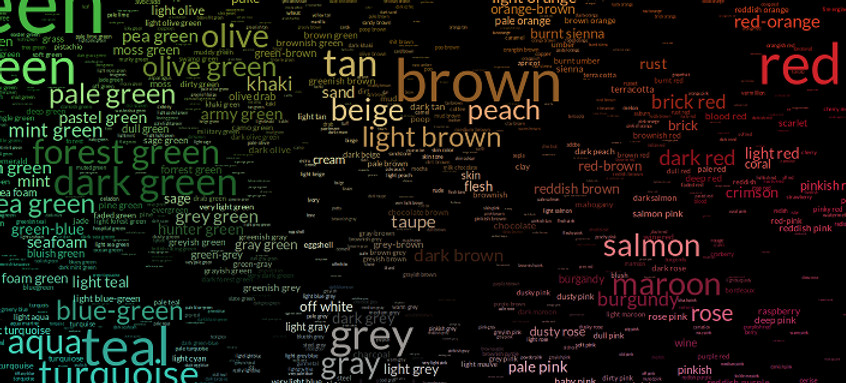
A while ago, Randall Munroe of xkcd.com ran a survey. It showed you a random color and asked you to name it. With the combined power of lots of people on the Internet, it collected over two million data points about what people call different RGB colors. He put up the results for anyone to download.
As linguistic data sets go, this one is awesome. If you want to know what colors are named, you could go the prescriptive route and ask Pantone for a definitive list. Or you could go the descriptive route, and just ask people in general, two million times.
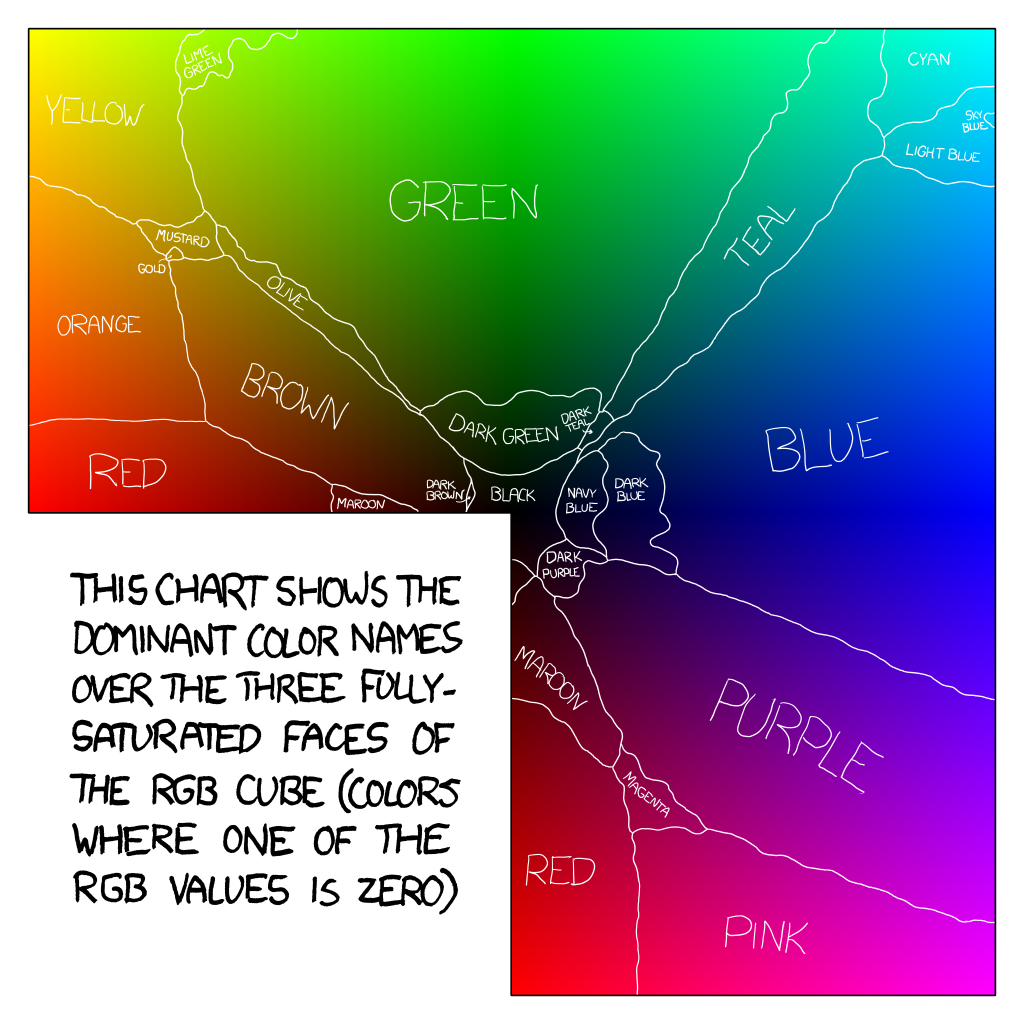
xkcd made some visualizations of parts of the space, like this diagram of the three saturated faces. That visualization is pretty cool, but it left me wanting more because that’s not nearly all the colors there are.
What if we could look through the color space, for example, and see all three dimensions of color at once? That usually doesn’t make a lot of sense, because the color space is solid. It doesn’t have gaps in it. You could try to make it semi-transparent or something, but then you’d just be seeing colors through other colors and it would be a mess.
But the space of color names does have gaps in it. Colors are a continuum, but color names are a sparse, finite set. So forget about the rest of the colors, because the colors that have names are interesting enough.
Word clouds
Probably our current favorite visualization at Luminoso is the word cloud. And when we say “word cloud”, we’re not talking about the amorphous thing you get out of something like Wordle. When we put words in different locations on the screen, those locations should mean something.
So we make sure to arrange them so that words with similar meanings stay together. Our ability to compare the meanings of words is based in part on our work with ConceptNet.
But enough about semantics, at least until later in the post. You’re here to see pretty colors.
The color cloud
So let’s apply the same kind of layout to the color names that came back from the survey. Instead of arranging words by similarity in meaning, we’ll just arrange colors by similarity in color. The result is a visually stunning, fractal-ish view of the world of color.
Note: This visualization does some pretty complicated text layout and it makes your browser do all the work. As far as we can tell, it works eventually in most browsers that aren’t IE, but it’s only going to be a pleasant experience in Chrome.
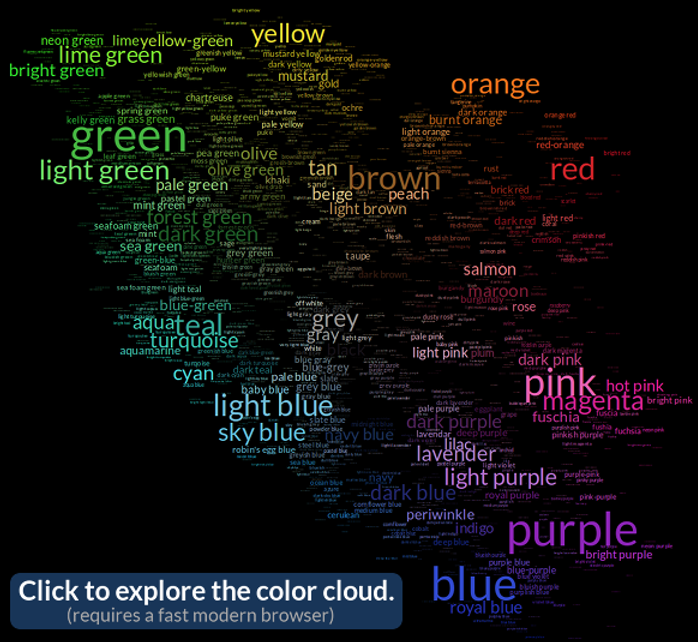
You might want to open that in a tab. We’re going to be referring back to it, and panning, zooming
, and rearranging it will be more fun than just looking at the cropped snapshots in this post.After the fold, we explain how we chose the colors, why they’re in the locations they are, and how we can sort out color names into “male-centric” and “female-centric”, or sort them semantically into “colors people like” vs. “colors people don’t like”.
Building the visualization
Here’s how this visualization works:
- We group together the responses by the color name that people gave.
- People were seeing a variety of colors when they gave those responses, of course, so we collected the set of colors and found the one closest to the median.
- Taking the median pretty much means we have to filter for color names that appeared at least three times. Despite the free-form responses, that’s actually a fair number of color names. You’d be surprised at the variety of names that appear three times, like duckweed, sky blue pink, deep purple (smoke on the water), 70s appliance green, and wednesday*. There’s also the fascinating pair blue enough and not blue enough, which were entered by entirely disjoint sets of people.
- If the average color in the set is too far from the median, throw out this color name. This eliminates most of the spam and the responses that don’t refer to specific colors, such as “wtf”. A few false positives sneak through when people happen to give the same non-color response to similar colors, such as lol idk and does this end. At this point, we have 18168 colors we can name.
- Put the more common colors in larger fonts — the area of a letter in a color name is proportional to the number of people who gave that response.
- Arrange the color names in a suitable two-dimensional projection, dodging the names of larger colors so they don’t overlap. By default, I arrange them by their “chromaticity”, a two-dimensional measurement that includes hue and saturation, while ignoring lightness. I’ll get to the color theory behind this at the end of the post.
* Why is Wednesday a color? Why is it a consistent color? Why did I suspect it would be orangeish even before I looked up its median color? Brains are weird.
Revisiting the differences between sexes
During the time Randall’s survey was collecting data, a Doghouse Diaries comic was posted about what women and men call different colors. Randall was collecting data about X and Y chromosomes in order to look at colorblindness, but it seemed an excellent opportunity to address this question, as well, so he did.
For this post, when I say “women” and “men,” or “female” and “male,” I’m referring to the answers xkcd survey-takers gave to the question about whether they biologically have a Y chromosome, if they responded. A discussion that’s recently very heated in Western society is that of sex vs. self-identified gender, and it’s something Randall got significant feedback about because of this survey. He wrote a followup post on the topic.
Luminoso, as a company, discussed sex and gender for approximately a week while writing this post. At this point, we are agreed that we all have different perspectives on the topic, but we don’t all agree as to which terms to use to refer to the individuals who selected “yes” to the question “Do you have a Y chromosome?”, or how to accurately report on cultural gender differences in color naming based on a biological question. It’s challenging and important to pay attention to this, despite it not being the topic of this blog post.
Back to the results of the survey and original analysis — Randall concluded that, somewhat regardless of sex, the respondents actually do mostly tend to agree on what to call the colors of the rainbow. Here’s his version of the diagram.
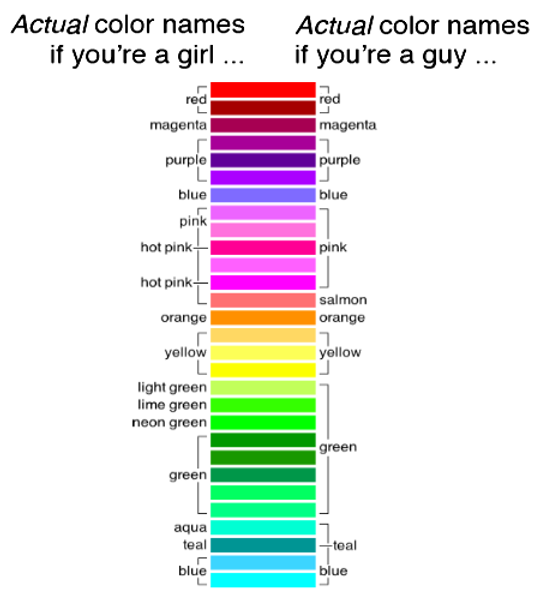
When we look back at the data, we find that the situation gets more interesting once we go below the rainbow surface. If you include less-saturated colors, you can find some pretty striking differences between the names given by men and women. To us, it looks more like the Doghouse Diaries version.
Randall searched for the “most feminine” and “most masculine” color names; you should be sure to read that part of the post because the results are hilarious.
I weep for my gender. – Randall Monroe
We don’t know exactly how Randall accounted for the number of total responses when making his top-5 lists. Certainly the “most feminine” colors aren’t just colors named by one female and zero males, for example.
We chose to apply a Student’s t-test, because it’s good enough for making beer and it’s good enough for us. Basically, the most masculine color names can be defined as the ones whose distribution of responses is most significantly above 62.8% male (the average male responses for the whole dataset), and the most feminine ones are the ones whose responses are most significantly above 37.2% female. (For this section only, we are omitting the 2.6% of responses from people who declined to indicate their chromosomes.)
The Student’s t-test is a measurement that increases not just as the percentage of male and female responses becomes more skewed, but also as the total number of responses becomes larger. You could say that this is designed to focus on the clearest differences, not the largest differences.
Also, this time, the top results will not be full of non-colors like “wtf” and “dunno”, because we’ve already filtered those answers out.
Here’s what you get:
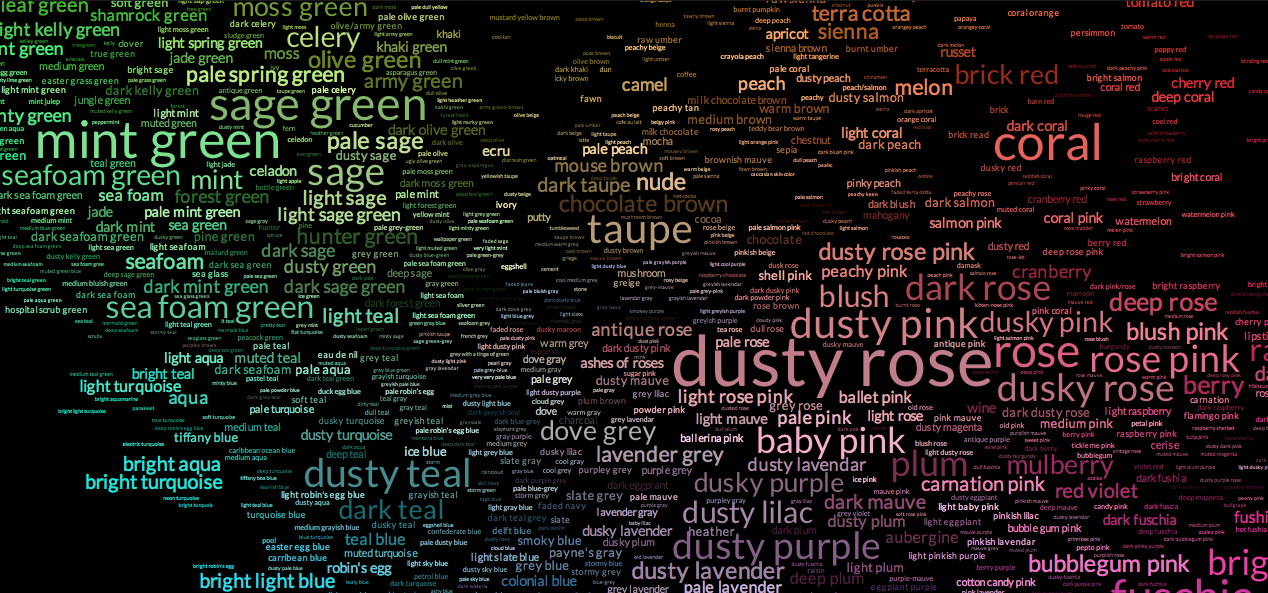
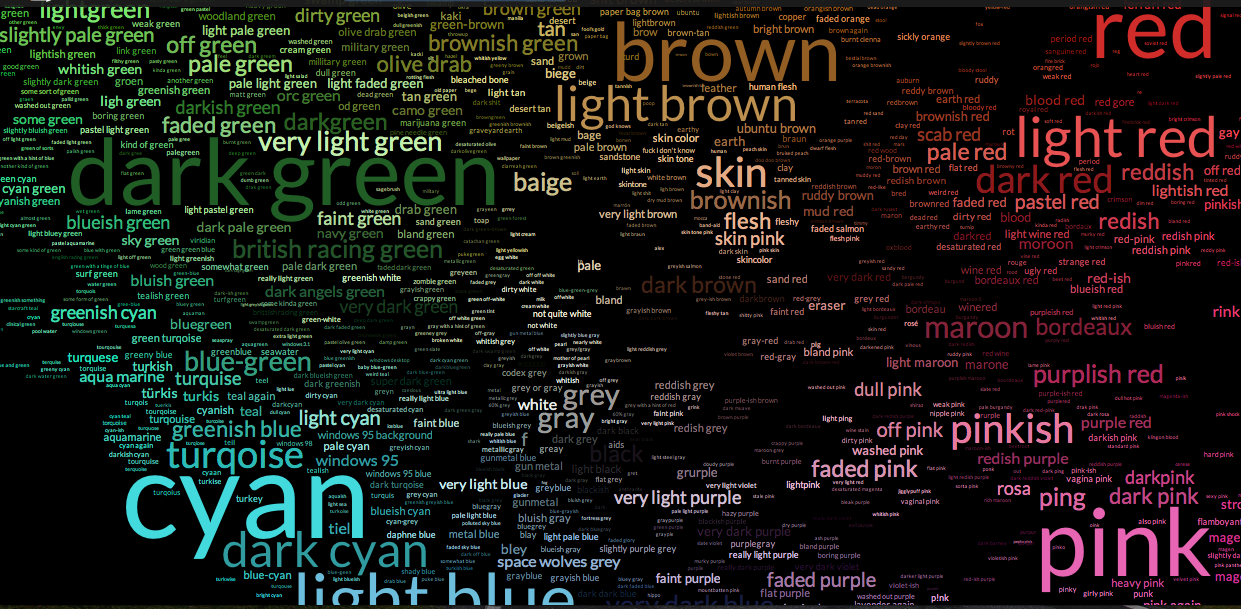
Now let’s generalize a bit, based on data that becomes more obvious when using the word cloud visualization.
If you want to follow along with the statistics: Below, when I show a color and its name, the values that follow, such as (female=6.7), are the results of the t-test. A number greater than 4 represents an effect that’s very significant, occurring by chance less than .1% of the time for most sample sizes. We’ll show results where the t-test value is less than 4.0 in either direction as “non-significant”. By running 18,000 t-tests simultaneously, we will encounter the green jelly beans problem in a few cases, but most of these results have t-test values that are so enormously high that they wouldn’t occur by chance even in 18,000 tests.
It’s true, males and females fundamentally agree on the rainbow. However, men really are more likely to use a simple rainbow color name such as red (male=37.4) or green (male=58.6), while women tend to at least add a descriptive word. In fact, the color name “green” (with no additional modifying words) has the single most significant difference between the sexes, with 71.6% of its 89494 responses being from men.
The main difference between the sexes is that, given a less-saturated color, men will often still call it by its rainbow color name, unless it’s desaturated enough that they call it a shade of grey like space wolves grey (male=7.1). Women more commonly add a descriptive adjective to distinguish shades, such as dusty red (female=6.7). In many cases, there is a separate name for the desaturated color that’s used much more by women, such as tawny (female=5.2), sage (female=27.3), taupe (female=28.1), or coral (female=34.3).
Spring green (female=50.7) is the queen of feminine colors, answered 2397 times by women and 767 times by men, but the less-frequent dusty rose (female=48.2) also has a solid claim on that title, with 1405 responses by women and 306 by men.
Besides the ubiquitous green, it’s apparently hard to get more masculine than cyan (male=54.1), answered 13336 times by males and only 3407 times by females. I’d theorize that’s because cyan is a nerdy technical color name that became popular because it’s a useful way to describe the color you get when you light up your RGB green (non-significant) and RGB blue (non-significant) phosphors equally, or the related color of ink that absorbs the most possible red light. While xkcd readers are nerds of all genders and sexes, it seems plausible that males would be the ones to predominantly choose this name in the presence of alternatives such as aqua (female=11.4) and turquoise (non-significant).
In fact, when we showed this post to xkcd employees, one such male employee was heard to exclaim, “That’s not cyan!” in response to the median cyan shade shown in this post, whereas one female Luminoso employee had to look the color up online to see what the fuss was about.
And yes, “baige” (male=11.1) is still men’s fault. We’ll focus more on misspellings in a bit.
Sorting names by what they mean
We mentioned that we can sort things out by their semantics, based on ConceptNet. So why not apply that to the color names?
Unlike what we usually do with Luminoso visualizations, we can’t use layout and color to convey these things, because we’re already using layout and color to mean what color it is. But we can show colors selectively, and emphasize colors by changing the font size.
One thing that came to mind is to focus on the “best” and “worst” colors, using semantic positivity and negativity associations that ConceptNet inherently utilizes. We created one view that emphasizes the colors with positive-sounding names, such as fiesta red, happy yellow, festive green, and sexy pink. Of course, we also made its opposite view, emphasizing the colors with negative-sounding names, such as sickening pink, prison grey, vomit, stupid purple, and screen of death.
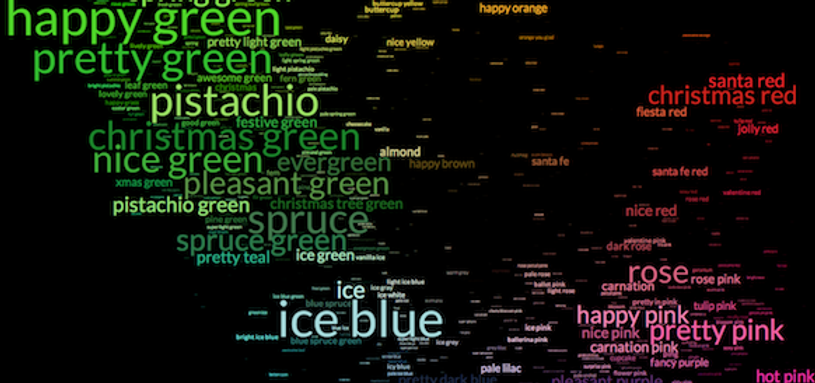

Yes, occasional results in there are weird. I haven’t yet looked into why ConceptNet is so fond of ice and so opposed to amber.
Goodness vs. badness isn’t the only kind of meaning we can identify based on concepts. A topic area that comes up a lot in color names is food. Lots of colors are named after edible things. Let’s make another view of color names that describe food:
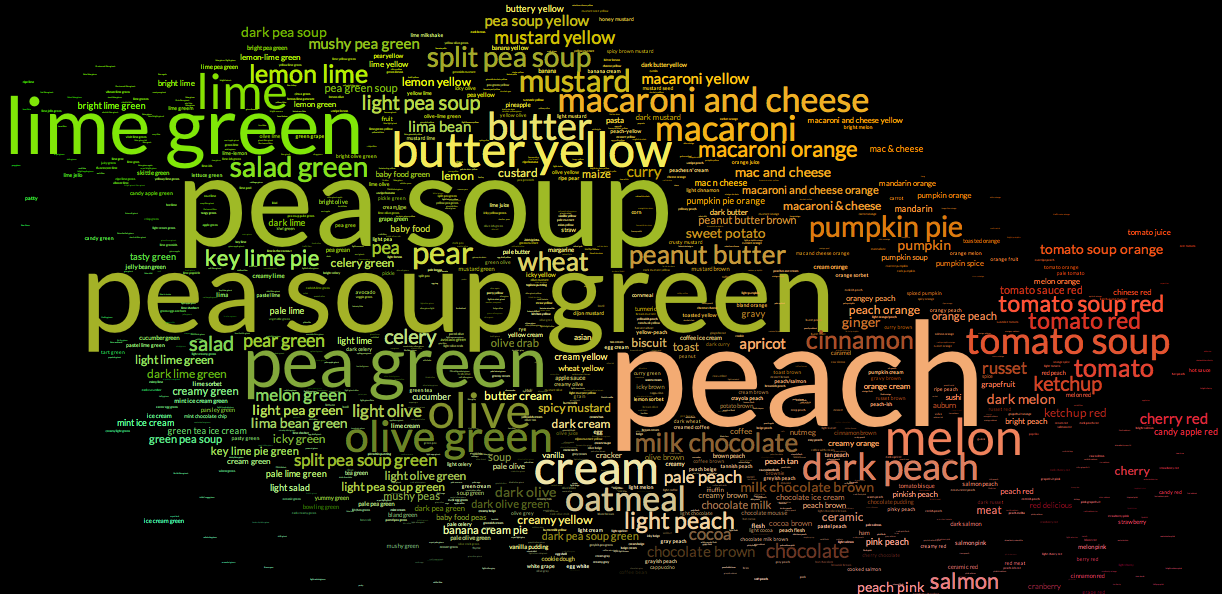
The interaction between food and color is interesting. Except for rare outliers such as grape and blueberry, this is the entire edible spectrum.
Misspellings and portmanteaus
Some of the color names in the results are ones that we could call “lexically creative”. They’re words you won’t find in a dictionary. In most cases, this is because we could also call them “misspelled”. Let’s focus on those for a moment.
Most wordlists out there have too many false negatives. For example, we shouldn’t flag the word “desaturated” just because the Official Scrabble Players’ Dictionary hasn’t heard of it. To find the most unusual words, we’ll compare to a wordlist we already use for other purposes, the Google Books 1-grams corpus.
Let’s say an unusual color name is one with a word that occurs so infrequently in print that it doesn’t make it into Google’s American English corpus, which is the largest of their 1-gram corpora that you can download. This means it occurs fewer than 40 times in over 100 billion words of American English. (Even British spellings show up there in statistically significant numbers.)
Here are the most common names that aren’t found in Google’s American English corpus, together with the number of times they appeared:
fuscia (2725), turqoise (2153), burgandy (1252), turquiose (716), turquise (597), pruple (576), chartruse (575), perriwinkle (573), purpley blue (572), blurple (548), purpleish blue (382), fuchia (373), fusia (372)…
So the most common of these are misspellings, especially misspellings of “fuchsia” and “turquoise”. Some of them use sensibly-spelled but informal adjectives, like “purpley” or “tealish”. My favorites are those that invent new color names as a portmanteau, such as blurple (548 responses) or grellow (67 responses). The most common example of a false negative where survey takers were smarter than Google Books was majorelle blue (21 responses).
So with this data, we can confirm what you’ve suspected ever since “baige” topped xkcd’s list of male-dominated colors. Male survey responders are somewhat more responsible for these unusual color words, which are mostly misspellings. Compared to 62.8% of responses from men overall, men are responsible for 68.1% of the unusual color words, and a t-test shows that as an extremely significant difference (male=25.8).
Color spaces
There is also some color theory behind the weird pointy shape we arrange the colors in, which might be good to explain at this time.
Computer screens inherently work with an RGB color space, building each color out of varying amounts of red, green, and blue light. When you pick an arbitrary color from the RGB space, there’s a good chance it’s going to be green. The green component of an RGB display is a frequency of light that the eye is very sensitive to, so green can easily drown out whatever else is going on in the color. Because the survey was sampling from RGB space, you can see this in responses such as green again (132 responses), still green (52 responses), and I’m sick of green (3 responses).
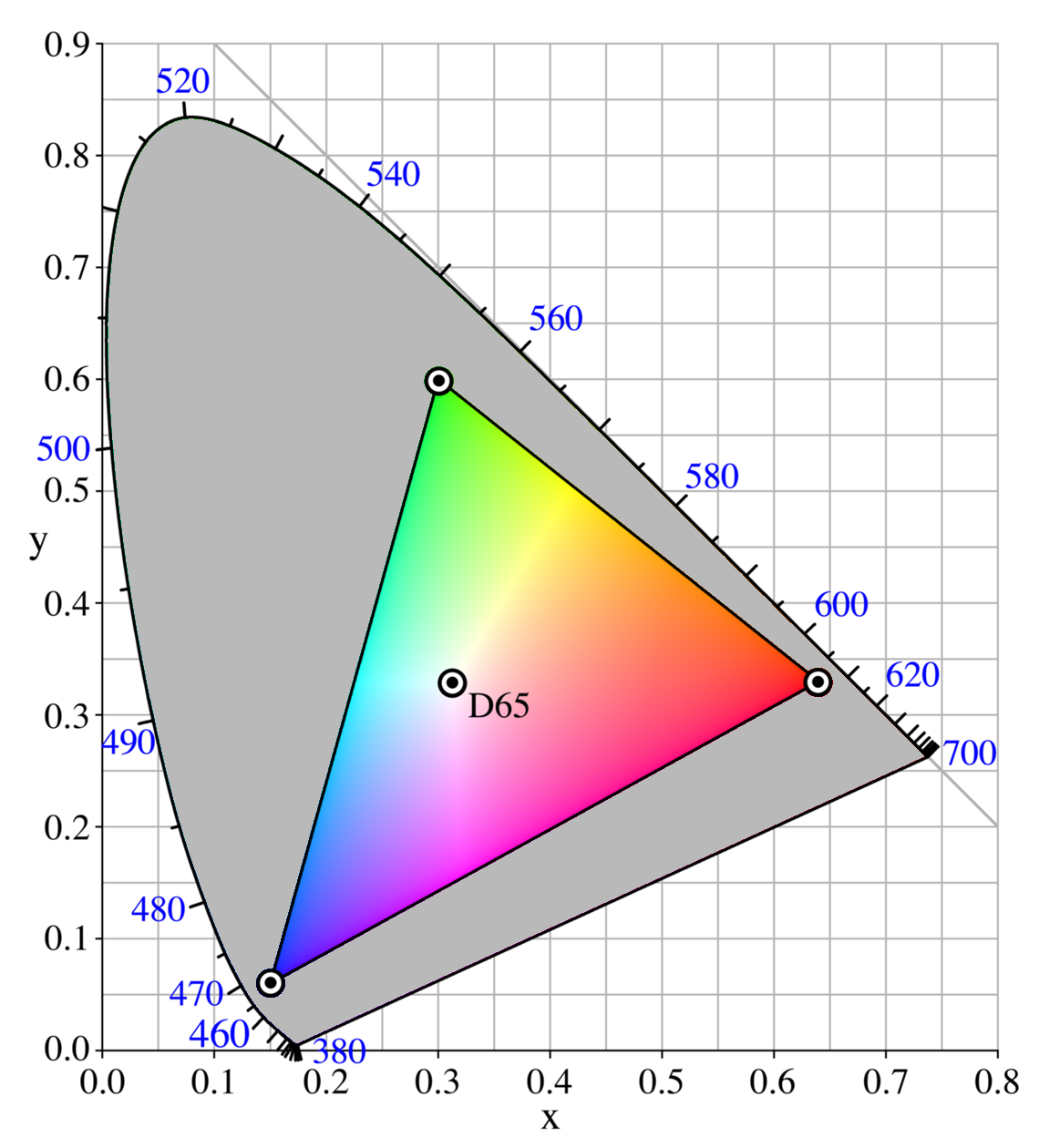
This diagram represents the space of physically possible colors (ignoring lightness), and it’s plotted linearly in terms of how the color is actually produced. The triangle represents the part of it that you can see on an RGB monitor. Look at the tiny little lines that cyan, magenta, and yellow get, compared to the vast expanses of green and shades of red. You’d be cramming a lot of interesting different color names together.
To represent the colors we actually care to distinguish, my preferred color space for showing a spectrum of colors is L*a*b*. (The asterisks mean its axes are nonlinear.) This space comes from measuring how people actually perceive color differences. A given distance in that space represents a corresponding difference in how people see the color.
L* is the component of luminance or perceived lightness, a* distinguishes red from green, and b* distinguishes yellow from blue.
Compared to a linear view of RGB space, this has the effect of squishing the greens and reds and creating much more room for all the different shades of purple, blue, and yellow. It also has the effect that, when you take the subset of the space a monitor can display, you get a pretty weird, wibbly-wobbly shape.
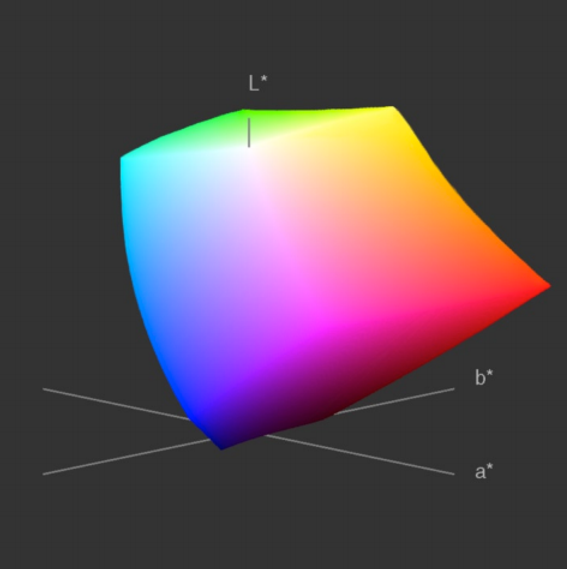
That’s the space we use by default for the visualization. You’re looking at it from the top down, seeing the a* and b* dimensions of the space. Colors with the same chromaticity but different luminance (L*), such as powder blue and navy blue, end up in the same place.
In the visualization, you can also choose from two more views that drop the a* or b* component instead. When you choose “red-green vs. lightness”, you’ll get an interesting-looking space where the blue vs. yellow component is ignored. It makes a kind of S shape because, again, you’re better at seeing the green your monitor produces than the red, so the greenest greens are perceived as brighter than the reddest reds.
Similarly, if you choose “blue-yellow vs. lightness”, the red-green component will be ignored. That one actually makes a pretty reasonable view of how red-green colorblind people will perceive differences in colors. It’s a space where brick red and forest green are almost the same, as well as teal and pink. The “red-green vs. lightness” space should be similar for blue-yellow colorblindness.
The “hue-saturation hexagon” is a view that’s like a traditional color wheel. I looked up the formulas for making a color wheel out of the RGB space, and I was not impressed by the step that basically says “now take the hexagon you got and distort it into a circle, because people like circles”. I left it as a hexagon, which seems like a more accurate representation of RGB.
In both the L*a*b* shape and the hue-saturation hexagon, you can see an interesting effect where the colors people agree on fall into particular bands by hue. Here I can see bands for red, orange, yellow, green, a continuum of teal-cyan-sky blue-azure-royal blue, then purple and magenta. Between many of these are noticeably sparse areas, for colors that people will tend to lump in with other colors more than they agree on a name for them. These “in-between” colors include ones we could call cerise, bittersweet, spanish orange, ecru, sage, and mauve.
And yes, I did just look up those names in the female version of the chart. I remember “bittersweet” from Crayola boxes, but I have never been sure what color “ecru” is.
I did the color space transformations for this visualization using python-colormath. I assumed that everyone’s monitors were calibrated to sRGB, though of course most monitors are not calibrated to anything in particular. I had to assume something. I could have tried to take into account the survey question that asked for your monitor’s color temperature, but hardly anybody answered that, and I don’t blame them.
Get the data
There’s certainly more we could have done with this data. A file that has all of the median colors in RGB could be a more convenient starting point than the original raw data, so here’s our version of the data in .json format. Interested? Have fun!